Research
Our group develops new theories and computational methods in the fields of quantum chemical calculations, molecular dynamics calculations, information science and their integration. By utilizing our technologies, we carry out unique applications and elucidate structures, reaction mechanisms, and functions of complex molecular system. In addition, we feed back the issues that emerge in application studies to the methodological development and establish fundamental techniques. We will lead the state-of-the-art of chemical computation & information through such a chain of activities.
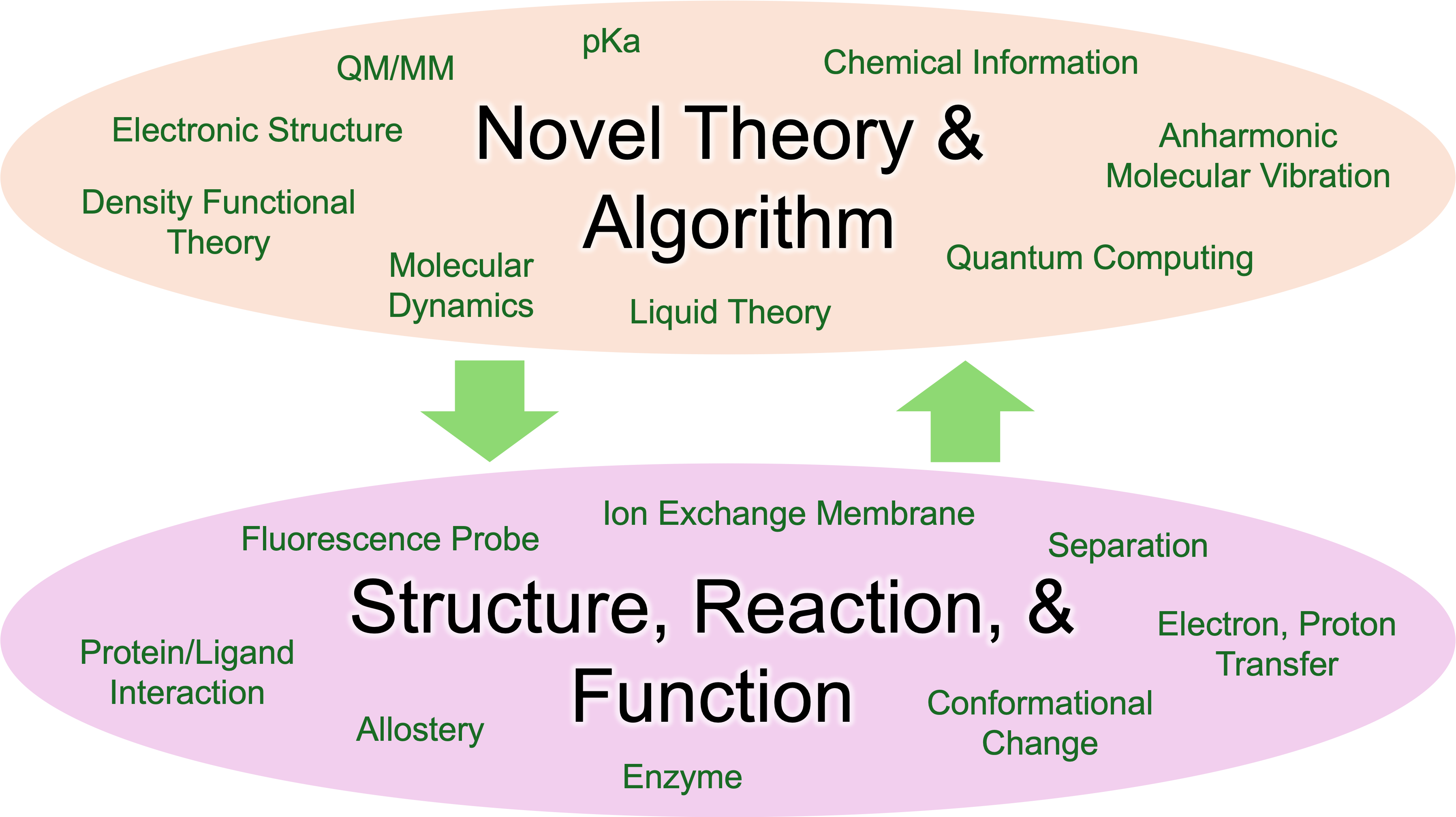
Novel theory and algorithm
1. Development of vibrational theories for complex molecules
Molecular vibrational analysis is one of the most powerful methods for understanding complex molecular interactions at the atomic and molecular level. The vibrational states of molecules are described by the vibrational Schrödinger equation. However, one of the big challenges is that the computational cost of solving this equation grows prohibitive with the number of atoms. We have developed a multiresolution method to generate anharmonic potentials using quantum chemical calculations [1] and a vibrational quasi-degenerate perturbation theory (VQDPT) [2] to efficiently solve the vibrational Schrödinger equation. These methods implemented in our original program SINDO, and distributed as an open-source, free program. An advanced method to calculate the vibrational spectra of proteins and macromolecules is now in progress.
- K. Yagi, S. Hirata, and K. Hirao, Theor. Chem. Acc. 118, 681-691 (2007).
- K. Yagi, S. Hirata, and K. Hirao, Phys. Chem. Chem. Phys. 10, 1781-1788 (2008).
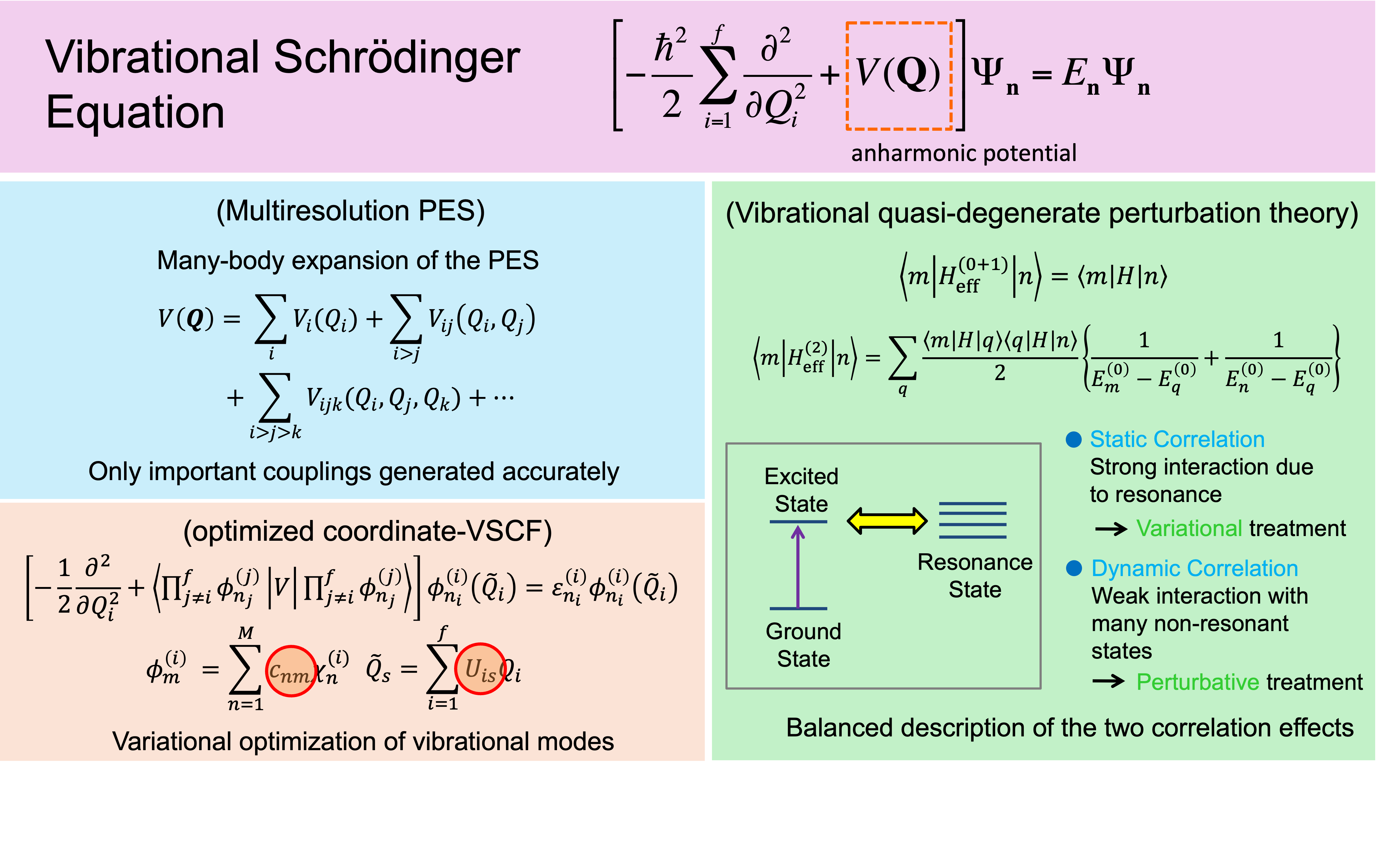
2. Acceleration of QM/MM molecular dynamics simulations
The QM/MM method is a multiscale method that treats chemically interesting subsystems with highly accurate quantum chemical (QM) calculations and the surrounding environments such as solvents and biomolecules with low-cost classical force field (MM). We have implemented the QM/MM method in the molecular dynamics (MD) simulation program GENESIS [1] and integrated it with the highly parallelized QM program, QSimulate-QM to achieve QM/MM-MD calculations that are 10 times faster than conventional ones [2]. Currently, an efficient free energy calculation combining the QM/MM method with the enhanced sampling method is under development.
- K. Yagi, K. Yamada, C. Kobayashi, and Y. Sugita, J. Chem. Theory Comput. 15, 1924-1938 (2019).
- K. Yagi, S. Ito, and Y. Sugita, J. Phys. Chem. B 125, 4701-4713 (2021).
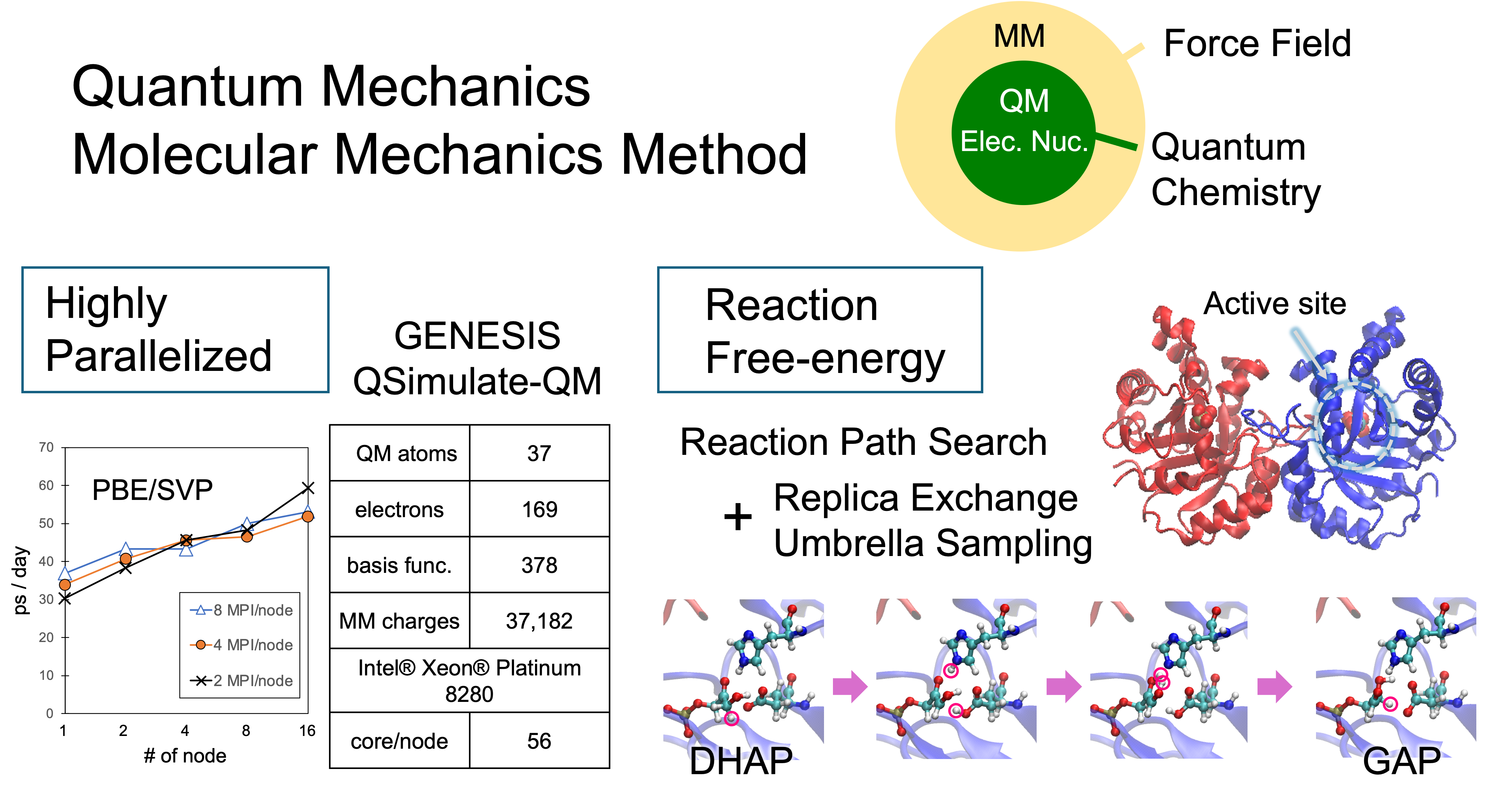
3. Development of density functional theory based on molecular information
Artificial intelligence (AI) and machine learning have brought revolutionary advancements to scientific research. In 2024, the developers of Rosetta and AlphaFold were awarded the Nobel Prize in Chemistry for their breakthroughs in protein structure prediction. Similar to the challenges in protein structure analysis, density functional theory (DFT), which is widely used in quantum chemistry calculations, also faces numerous parameterization issues. In our laboratory, we have developed a methodology to determine parameters in theoretical constraints, independent of experimental data or high-level ab initio calculations [1]. This approach is further enhanced by combining it with machine learning-based fitting. We are currently developing processes to construct new functionals aimed at achieving higher accuracy.
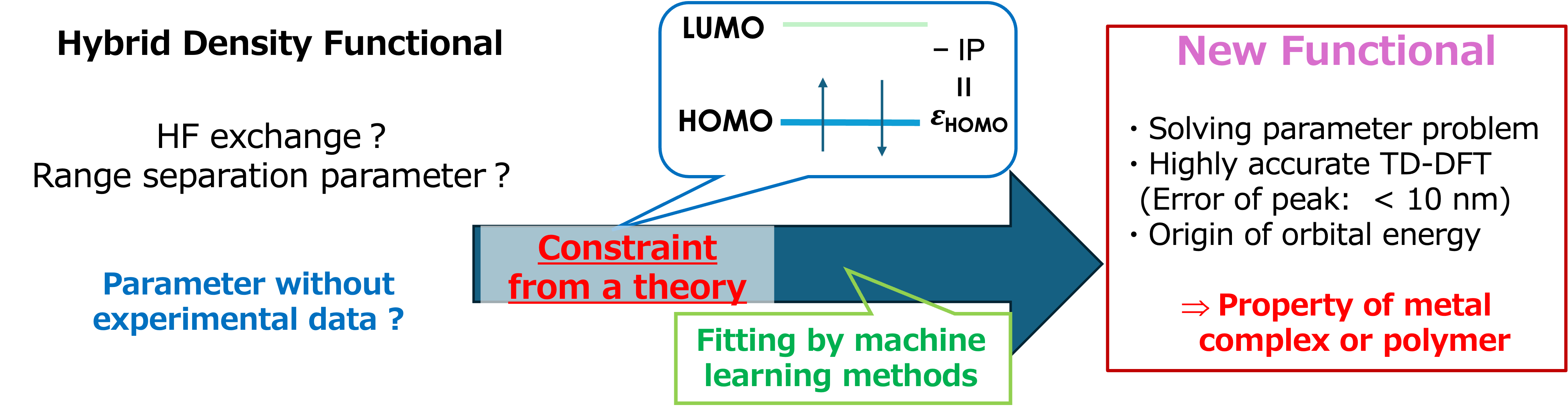
Revealing the Structure, Reaction and Function
4. Elucidation of the molecular mechanism of functional polymer membranes
Functional polymer membranes, which selectively permeate and separate molecules and ions, are important materials for energy and environmental issues. The improvement of their performance requires an understanding of molecular mechanisms at atomistic levels. However, little is known about their key processes, namely, how the interfaces and amorphous portions of polymers are structured and how small molecules move in such an environment. We have utilized our original techniques, weighted average method and molecular vibration analysis, to elucidate the mechanism of water molecule permeation in reverse osmosis (RO) membranes [1]. Currently, research projects for ion exchange membranes and electrolyte membranes are in progress.
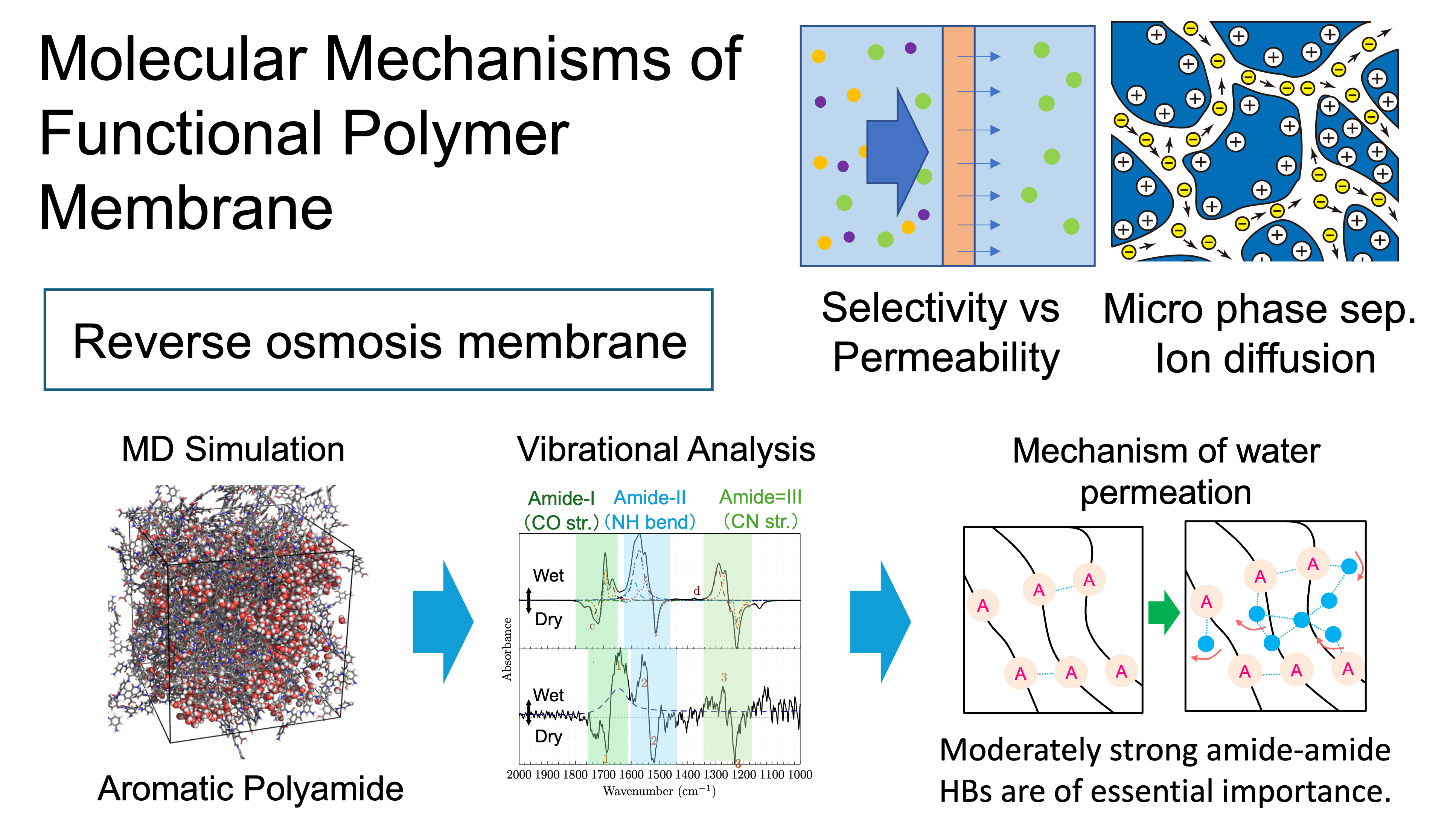
5. Elucidation of interactions between proteins, small molecules, and light
Proteins achieve biological functions by undergoing various conformational changes in vivo. In many cases, the driving force is events such as binding of small molecules (substrates) and light absorption. We use QM/MM-MD calculations to track chemical reactions after substrate binding and/or photo-absorption and elucidate their molecular mechanisms. Recently, in collaboration with synthetic organic chemistry, we designed a fluorescent probe that undergoes photoemission only when it binds to a target protein [1]. Currently, further improvement is being explored by utilizing informatics tools. Projects on enzymatic reactions and light-driven proteins are now ongoing.
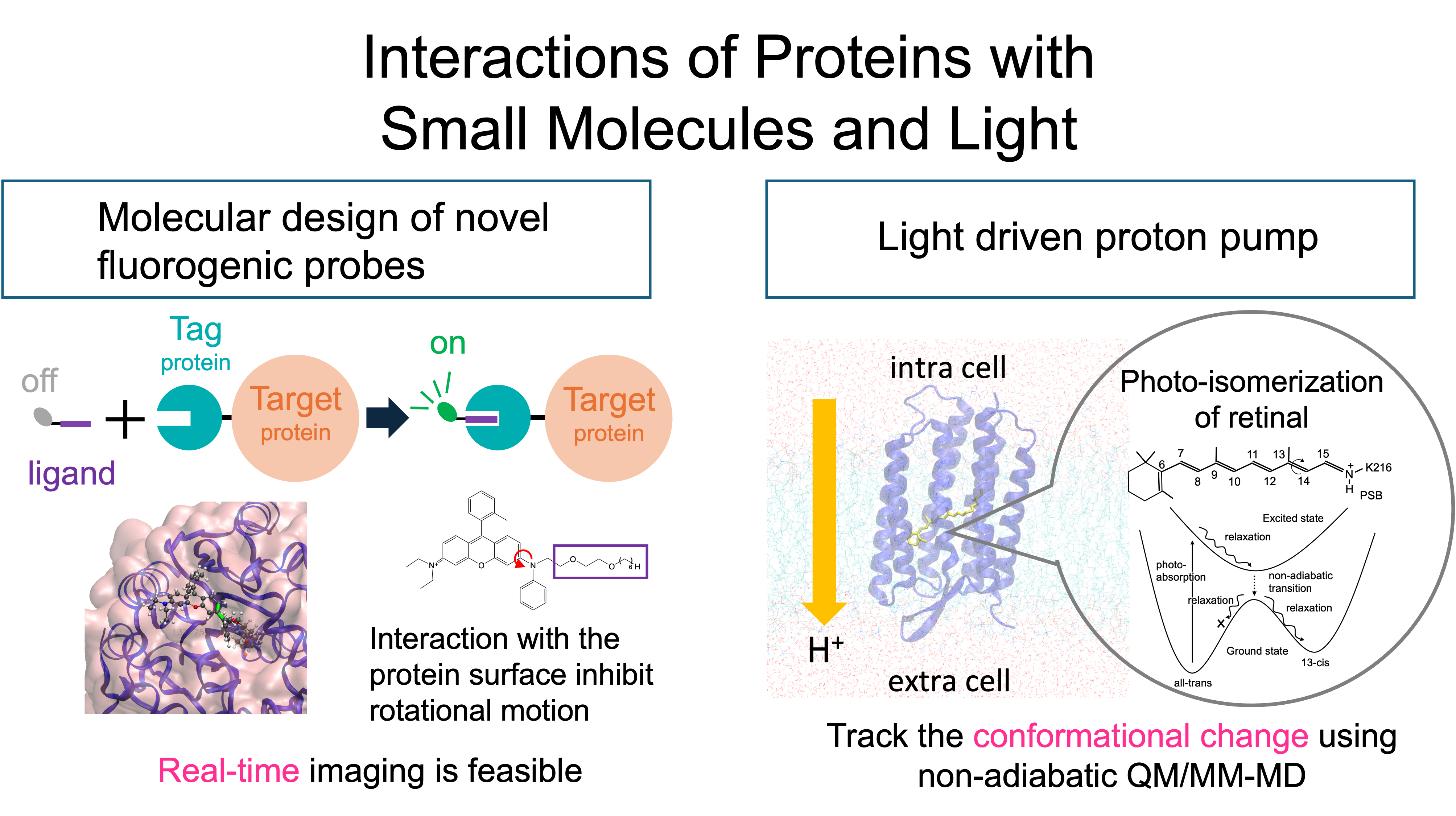
6. Calculations toward powerful molecular generative AI based on quantum chemistry
Our laboratory aims to predict the highly complex properties of materials, such as polymers and metal complexes, from the perspective of quantum chemistry calculations. For instance, in novel materials like organic light-emitting diodes (OLEDs) and organic thin-film solar cells, an accurate evaluation of charge-separated states is crucial for performance improvement. We have implemented density functional theory that describes charge-separated states and applied it to organic thin-film solar cell systems to analyze the likelihood of charge separation [1]. We have also computed and proposed electron transfer pathways for biological molecules based on pKa and redox potentials [2]. By accumulating these computational data, we aim to develop molecular generative AI in the future.
- T. Fujita, T. Matsui, M. Sumita, Y. Imamura, and K. Morihashi, Chem. Phys. Lett. 693, 188-193 (2018).
- S. Maekawa, T. Matsui, K. Hirao, and Y. Shigeta, J. Phys. Chem. B 119, 5392-5403 (2015).
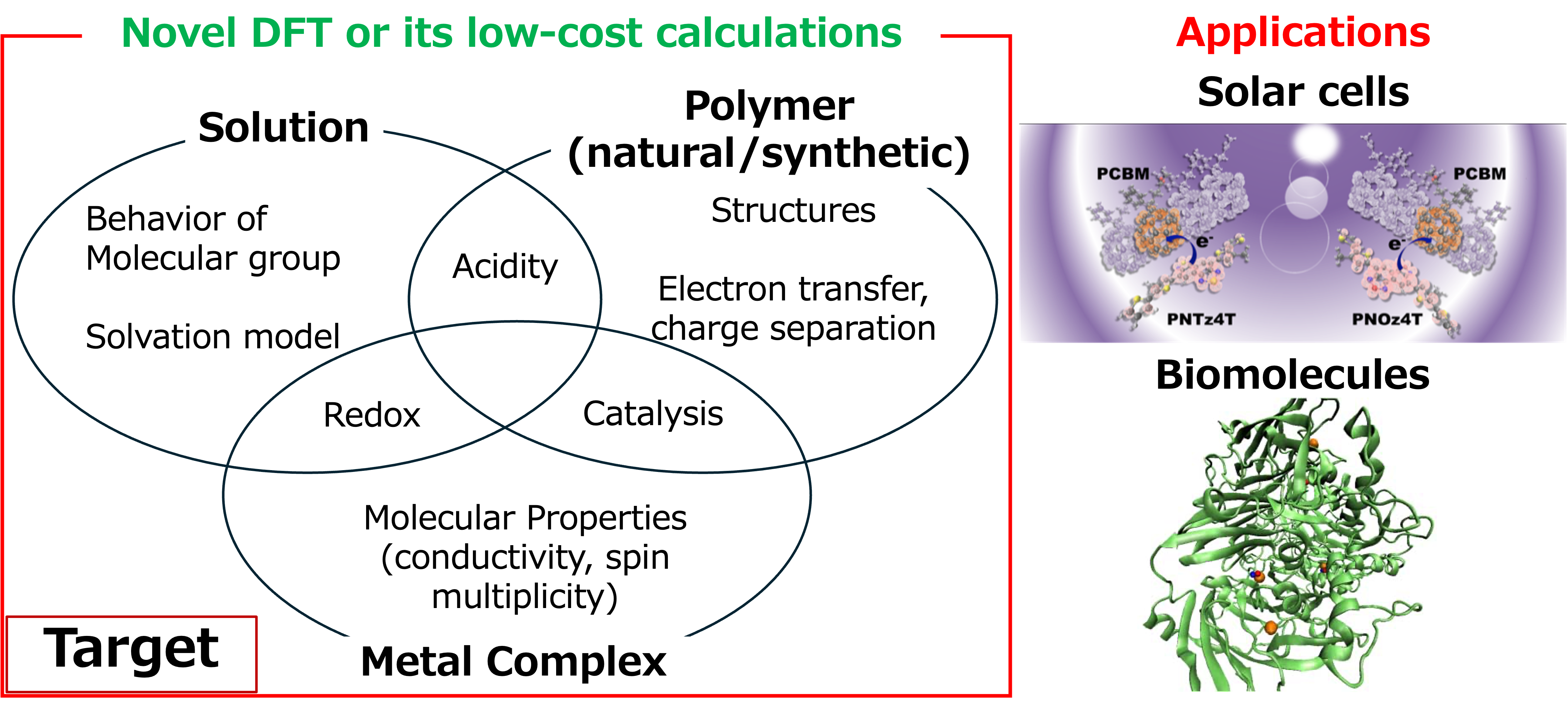